Technology 4.0 is an essential part of our lives that makes our day-to-day tasks much easier without us even realizing it. This term includes all the digitalisation and automation processes that we see in the industry and also in our daily lives, finding among them Big Data, artificial intelligence, robotics, the Internet of things, and virtual and augmented reality. All these technological developments are changing our lives so deeply that this situation has been called the fourth digital revolution, as we explained two years ago in this same blog (see post here).
After having dedicated most years of my career to the research in plant physiology and agronomic engineering, one of the most fascinating and promising applications I find that the digital revolution offers to this field is precision farming, also called agriculture 4.0. There is no doubt that eating is one of our everyday activities that we cannot go without, but not everyone is aware of its great importance. In the recent health crisis caused by the spread of the coronavirus, the agri-food sector has demonstrated its strength and strategic role throughout the entire production chain: from farmers, livestock breeders and fishermen to supermarkets and food stores. The sector has been committed to digitalisation for years, and now this has become a necessity if they want to keep its competitiveness in this global crisis that has followed the pandemic.
Precision farming is based on the collection of data that influence crop productivity, such as environmental factors, soil, orientation and orography of the land, etc. All this data are later integrated with advanced software to develop predictive models. These models allow the determination of the optimal dose of irrigation and fertilizers, and can accurately predict the danger of pests and diseases. This translates into a great saving and, at the same time, a great environmental benefit as the amount of water and chemical products applied is drastically reduced. But how is all the data collected and recorded?
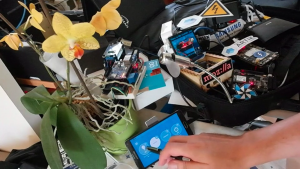
Figure 1: an example of a simple humidity sensor connected to the Internet via an Arduino board. Collected data can be viewed in real time in the mobile application, as well as the history. Photo credit: Vimeo.
There are different devices and technologies, including drones that capture images of crops with different types of cameras. RGB (red, green, and blue) cameras capture images visible to the naked eye and allow the detection of areas with little vegetation, waterlogging, etc. Multi-spectral cameras capture images of light spectra that cannot be seen by the human eye. Among other things, they can measure the amount of light absorbed and reflected by plants within the visible and non-visible spectrum, the amount of active chlorophyll, and the infrared radiation emitted by plants. All these images are processed after the drone’s flight or, more commonly, sent to a computer platform in real time thanks to a built-in Arduino plate. An Arduino [1] is an open source programmable miniature computer board that, among other uses, can connect devices to a WiFi network and send data to the Cloud [2] or to a computer platform that records it (this is called the Internet of Things (IoT) [3]). Through mathematical formulas, we can process drone images to know the plant vigour, to measure the amount of active chlorophyll and photosynthesis, to know if a plant is well hydrated or water stressed, if it suffers from any disease, etc. In addition to drones, weather stations and soil moisture sensors are also used while being connected to the Cloud by Arduino or a similar IoT system, which records the data and sends them to a centralized computer system. Figure 1 shows a simple moisture sensor that transmits the moisture data from the pot with the orchid to a mobile application by using an Arduino plate. The following video provides a better understanding of how it works:
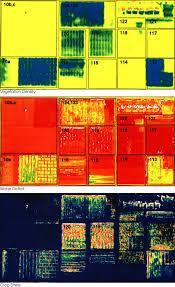
Figure 2: three types of images of the same plot in Arizona, obtained by a NASA aircraft by combining different cameras. Top picture shows the vegetation index (the plant density), the areas with water deficit are shown in the middle picture, and, at the bottom, you can see the plants that are very stressed due to disease or lack of nutrients. Photo credit: Wikimedia.
Depending on the size of the plot and the needs of each farmer, different combinations of devices, technology, and software are used. For large areas, such as those of some wine cellars or oil producers, this method is an indispensable optimization tool to effectively manage all growing areas, especially using drones. For example, it can happen that a plot or area of the plot has fewer hours of sunshine and accumulates more rainwater, and this is captured by the drone’s cameras and by the weather station. Figure 2 shows three types of images taken in the same field and in the same area. By correlating these climatic data with historical data on the appearance of pests and diseases, mathematical models based on artificial intelligence are developed to predict that in that area, and under those variables, there is a greater incidence of certain fungi and diseases, which always appear at a certain time of the year. These are “machine learning” algorithms: the system learns through the input data. The prediction software that includes these algorithms monitors in real time all the parameters that can compromise the productivity of the crop or the quality of the final product. This software is accessible through the web or mobile devices. Thanks to this information, the farmer can take preventive measures so that diseases do not affect the crop, such as applying a higher dose of phytosanitary products in that specific area. It is also possible to accurately predict the demand for irrigation, the optimum time for sowing and harvesting, and productivity; allowing the farmer to act quickly, efficiently, and in an environmentally friendly way.
On small plots of land where the use of drones is not feasible, predictive models can also be developed by combining weather stations and environmental and soil sensors (e.g., measuring humidity, pH, nutrient content, etc.). However, we must bear in mind that precision agriculture is still not very widespread on small farms, either because of the price, lack of knowledge, or the poor connectivity that still exists in some rural areas of Spain. The startup I am working on now, UpIntelligence [4], aims to bring this technology to small farmers and small food industries, listening to the needs of each one and proposing simple and tailor-made solutions.
It is estimated that by 2050 the world’s population will reach 9.8 billion people [5]. To feed this entire population, the agricultural industry will have to produce 70% more food, using only 5% more arable land than is available today. This, coupled with the current dramatic context of climate change, is an almost impossible challenge for today’s global agriculture, and European regulations already exist governing the use of plant protection products to reduce their toxic effects on the environment. For all these reasons, the implementation of precision farming, including the use of digital technologies and Big Data, will become an obligation [6].
In short, the digital transformation of the countryside at all scales and throughout the production chain will be increasingly necessary if we are to ensure that what we eat every day is sufficient for everyone, sustainable and, above all, of good quality.
* * *
By Olaya Muñoz Azcárate (@OlayinaBio), PhD in Biology. Project manager for agrifood sector. Up Intelligence. Gijón (Spain)
More information:
- Information about Arduino. Source: Arduino.
- Information about the cloud. Source: Cloudflare.
- Information about the Internet of Things. Source: Wired.
- Startup UpIntelligence.
- Post about “World population prospects: the 2017 revision”. Source: United Nations.
- Photo credit for featured image: excerpted from a US GAO report. Retrieved from Wikipedia.
- Information about precision agriculture. Source: Wikipedia.